Deep Learning Approach for Volume Estimation in Earthmoving Operation
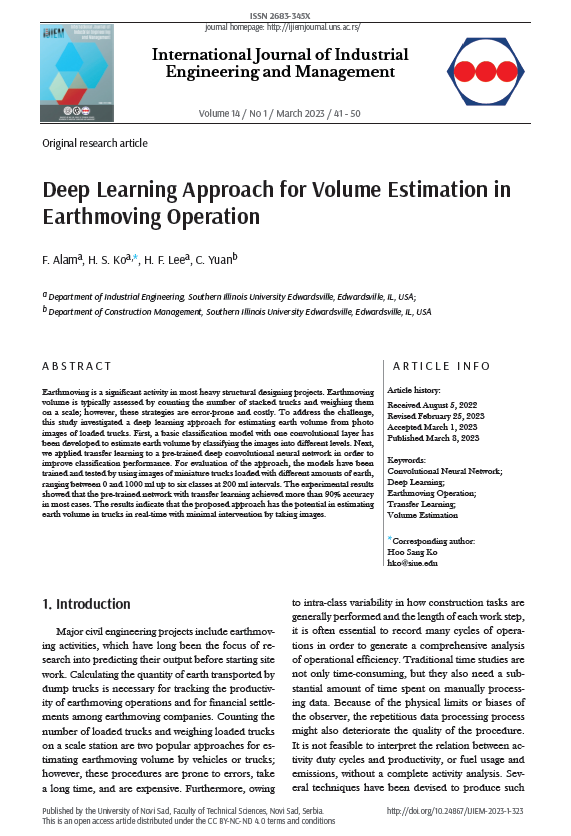
Published 2023-03-30
abstract views: 87 // FULL TEXT ARTICLE (PDF): 0
Keywords
- Convolutional Neural Network,
- Deep Learning,
- Earthmoving Operation,
- Transfer Learning,
- Volume Estimation
How to Cite
Copyright (c) 2023 International Journal of Industrial Engineering and Management

This work is licensed under a Creative Commons Attribution 4.0 International License.
Abstract
Earthmoving is a significant activity in most heavy structural designing projects. Earthmoving volume is typically assessed by counting the number of stacked trucks and weighing them on a scale; however, these strategies are error-prone and costly. To address the challenge, this study investigated a deep learning approach for estimating earth volume from photo images of loaded trucks. First, a basic classification model with one convolutional layer has been developed to estimate earth volume by classifying the images into different levels. Next, we applied transfer learning to a pre-trained deep convolutional neural network in order to improve classification performance. For evaluation of the approach, the models have been trained and tested by using images of miniature trucks loaded with different amounts of earth, ranging between 0 and 1000 ml up to six classes at 200 ml intervals. The experimental results showed that the pre-trained network with transfer learning achieved more than 90% accuracy in most cases. The results indicate that the proposed approach has the potential in estimating earth volume in trucks in real-time with minimal intervention by taking images.
Article history: Received (August 5, 2022); Revised (February 25, 2023); Accepted (March 1, 2023); Published online (March 8, 2023)